Introduction
Interest in machine learning and artificial intelligence (AI) has increased over recent years, and new techniques such as deep learning (DL) have achieved impressive results in multiple diverse fields. Modern image recognition techniques have proven to be very capable when applied to the increasingly available medical datasets, with algorithms performing at or above the level of physicians at a number of specific tasks such as skin cancer classification, pneumonia detection on chest x-ray, and arrhythmia detection on electrocardiogram.1–3 In addition to the advances in computer vision, there has also been significant progress in prediction from other types of datasets. AI algorithms were recently applied to over 200,000 electronic health records (more than 42 billion data points) with significant noisy and missing data yet were able to accurately predict in hospital mortality, 30-day mortality, and unplanned readmission rates.4 AI algorithms are also able to identify subtle changes and patterns in vital sign monitoring data, providing early warning alerts for sepsis and patient deterioration.5,6 AI technologies have already demonstrated their potential to impact almost all areas of healthcare, however research in this area appears to have been taken up by different specialties with varying enthusiasm. To the authors knowledge there is currently no data quantifying this discrepancy. This bibliometric analysis compares the contribution of different medical specialties to AI research over the last 30 years.
Materials and Methods
The entire Web of Science database was searched on 23rd of May 2018 for the terms “artificial intelligence”, “machine learning” or “deep learning” (Box 1). Results were limited to articles, proceedings papers, or reviews. The Web of Science database is organised in line with the Organisation for Economic Co-operation and Development (OECD) category scheme. As the Web of Science database contains multiple disciplines, results were refined to the Web of Science categories mapped to the OECD 3.02 Clinical Medicine schema (containing over 12,000 journals/conference proceedings) between the year 1988 and 2018. All categories mapped to the OECD 3.02 Clinical Medicine subcategory were searched to achieve methodological integrity, reproducibility, and to optimise search sensitivity. Full references with citation data up to 23rd May 2018 were downloaded for all results, and duplicates were removed. The list of medical specialties incorporated 44 medical specialties officially recognised in Australia, United States, United Kingdom, or the European Union.
A search tool was created to query the author affiliation data in Microsoft Excel and Microsoft Word. Multiple key terms (including spelling variations where appropriate) were mapped to each listed specialty. A category for author affiliation with computer science departments was also used to assess inter-disciplinary collaboration. This key terms list was created through use of the Scopus Address Abbreviation Guide, word frequency analysis of the author affiliation data (manual review of the top 5000 words looking for appropriate key terms), and iterative review of terms within the affiliation data. The goal of the search tool was to maximise sensitivity in retrieving results with affiliation data showing contribution was made to the article by a listed specialty.
Article affiliation data were searched using the created search tool, and each result was manually reviewed. False positive results were removed or reassigned to the appropriate specialty category. If the search result was appropriate then the article was labelled as belonging to the corresponding specialty. If an article contained multiple hits for a key term it was only counted once. If an article was returned for key terms from two different specialties it was counted as both specialties.
Analysis was performed in Microsoft Excel for total count of articles per specialty over the time period, changes in specialty publication over time, inter-specialty collaboration as measured by articles that were labelled as two or more specialties, impact as measured by article citation rate, and top publishing journals. P-values were calculated using the two-sided chi-squared test for proportions. Secondary analysis was performed to assess collaboration of medical specialties with computer science or computer engineering departments.
Results
Initial database search returned 3942 results, with 3937 unique results once duplicates were removed. Medical specialty analysis was performed using the developed search tool, returning 2381 articles from 789 different journals. Radiology published significantly more articles than other top specialties (p < 0.001), being involved in 783 articles (33% of returned total), followed by psychiatry (406 articles), neurology (287 articles), paediatrics (239 articles), and cardiology (166 articles). Overall, 42% of articles were a result of a collaboration of two or more medical specialties, and almost a third (30%) of articles involved collaboration with computer scientists or computer engineers (Table 1). There were notable collaborations between radiology, psychiatry, and neurology, and also between paediatrics and psychiatry (Table 1). Annual publications for the top five publishing specialties has increased over the last 5 years (Fig. 1). There was a significant increase in yearly publication from 1988 to 2018 (p < 0.001). Total publications increased from 1 in 1980 to 1004 in 2017 (Fig. 2). Secondary analysis showed radiology was also the specialty that collaborated most often with computer scientists and computer engineers. Radiology also achieved the highest number of total citations (12,392) followed again by psychiatry and neurology (Table 2). Geriatrics achieved the highest median citations (10), followed by psychiatry (6), immunology (6), and genetics (6) (Appendix).


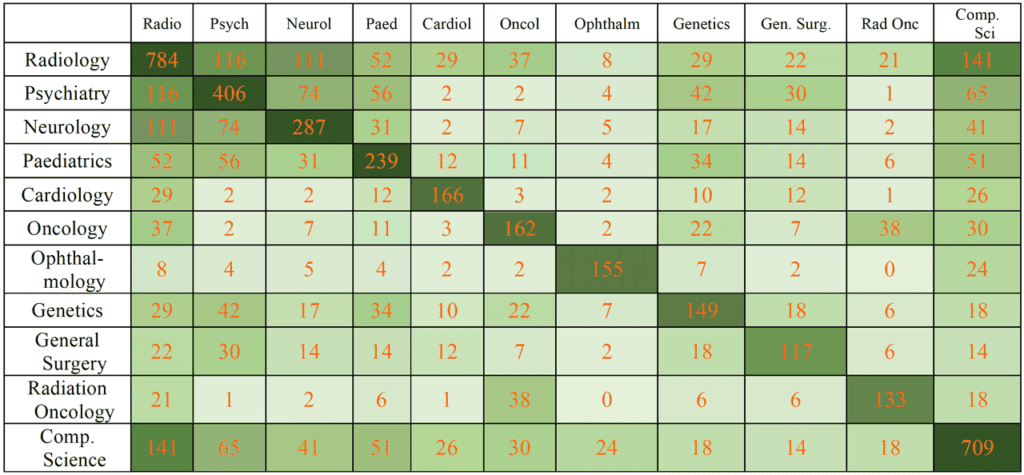
Discussion
There is significant and increasing interest in the use of AI in healthcare, which is in line with the general increase in AI capability over recent years.7 It is perhaps not surprising that radiology leads in involvement in AI related publications, since imaging itself contributes to so many specialities and an abundance of data is generated. Given the large volume of data, one could in fact argue that there is a paucity of radiology-based outcomes articles in the literature. We hypothesize that a large volume of the information encapsulated in medical images is currently unused, and can be “unlocked” from the human observer with learning algorithms. Moreover, there have been significant recent improvements in computer vision, with the state-of-the art accuracy increasing from 71.8% in 2010 to 97.8% in 2017 for the popular annual ImageNet computer vision challenge.8 Medical imaging datasets are often large and well labelled. This is a good match for modern computer vision and deep learning techniques, demonstrated by the significant collaboration between radiologists and computer scientists. There also appears to be a large amount of interest in the use of AI technologies in neurology and brain imaging, where deep learning again shows promise in decoding complex relationships. At the same time, it will become a priority to develop algorithms capable of testing hypotheses from less structured and unannotated data sets.
Our results show significant inter-specialty collaboration and interdisciplinary collaboration. Physicians are able to provide subject matter expertise and develop appropriate and useful clinical questions but are unlikely to have the computer science or programming skills required to develop and deploy state-of-the-art AI technologies. Indeed, as AI becomes more integrated into the practice of medicine it is likely that this research collaboration will become increasingly important.9 Radiology appears to be one of the first medical specialties to confront the disruptive potential of increasingly capable AI technology. There has been ongoing discussion concerning the future of the specialty with some suggesting that machine learning the “ultimate threat” to radiology, having the potential to replace radiologists altogether.10 Others argue that AI will be of great benefit to radiologists by “increasing their value, efficiency, accuracy, and personal satisfaction.”11 Despite the significant interest and volume of publications, use of AI in clinical radiology is still in its infancy.12 It seems most likely that, at least for the foreseeable future, AI technologies will augment rather than replace radiologists and physicians in general. We also opine that “more is not better”; the number of radiologists and physicians in general should react to the technologies that improve the quality of life for patients. These adjustments are largely driven by technologies. In the case of radiology, advanced imaging modalities (CT and MRI being the most obvious examples) led to a spike in the number of specialized physicians. If AI in turns leads to more efficiency, then the number of radiologists needed in healthcare could decrease.
Total citations for published articles | Mean number of citations for published articles | Median citations for published articles | |
---|---|---|---|
Radiology | 12392 | 15.8 | 3 |
Psychiatry | 6826 | 16.8 | 6 |
Neurology | 5065 | 17.6 | 4 |
Genetics | 2986 | 20 | 6 |
Paediatrics | 2885 | 12.1 | 3 |
Oncology | 2525 | 15.6 | 2.5 |
Ophthalmology | 2049 | 13.2 | 5 |
Urology | 1946 | 24.3 | 5.5 |
Cardiology | 1629 | 9.8 | 3 |
General Surgery | 1513 | 10.4 | 2 |
Neurosurgery | 1438 | 12.4 | 2 |
Radiation Oncology | 1390 | 10.5 | 2 |
Immunology | 1307 | 20.7 | 6 |
Public Health | 1226 | 10.5 | 3 |
Geriatrics | 919 | 36.8 | 10 |
Gastroenterology | 911 | 13.4 | 3.5 |
Respiratory Medicine | 895 | 19 | 5 |
General Medicine | 846 | 9.5 | 3 |
Infectious Disease | 773 | 25.8 | 4 |
Nuclear Medicine | 749 | 9.5 | 2 |
Though effort was taken to ensure accurate representation of the literature, this study has limitations. This was a bibliographic analysis of a single large database and it is possible that relevant journals may not have been indexed and so not included in the results. There also may be bias inherent in the database and the journals mapped to the OECD clinical medicine schema. Due to the very large number of specific machine learning, deep learning, and artificial intelligence techniques, the search strategy was developed using only very broad terms. Text analysis was limited to departmental affiliation data and may have missed relevant results that did not have departmental data or were labelled outside the scope of the search tool. The articles returned by the search tool were not read in full and there is a chance that some irrelevant results may have been included. Significant effort was invested in developing and testing the search tool, however specialty categories were not all-encompassing, and different locations in the world recognise and group specialties differently. It is likely that the search tool and search strategy underestimate the volume of AI related publication in all specialties and underestimate the involvement of computer scientists/engineers. All paediatric medical specialties were grouped under a single label which likely accounts for the high amount of publications. There is also departmental ambiguity in some results, with multiple specialties being listed under the same department (e.g. anaesthesia and intensive care). The specialties specific role in the study was not assessed, and contribution towards the AI component of the study may have been variable.
TOPIC: ("machine learning") OR TOPIC: ("deep learning") OR TOPIC: ("artificial intelligence") Refined by: SPECIALIST CATEGORIES: (RADIOLOGY NUCLEAR MEDICINE MEDICAL IMAGING OR CLINICAL NEUROLOGY OR PSYCHIATRY OR ONCOLOGY OR NEUROIMAGING OR CARDIAC CARDIOVASCULAR SYSTEMS OR MEDICINE GENERAL INTERNAL OR SURGERY OR OPHTHALMOLOGY OR CRITICAL CARE MEDICINE OR GASTROENTEROLOGY HEPATOLOGY OR ENDOCRINOLOGY METABOLISM OR RESPIRATORY SYSTEM OR PERIPHERAL VASCULAR DISEASE OR UROLOGY NEPHROLOGY OR HEMATOLOGY OR ANESTHESIOLOGY OR DERMATOLOGY OR GERIATRICS GERONTOLOGY OR OBSTETRICS GYNECOLOGY OR ORTHOPEDICS OR AUDIOLOGY SPEECH LANGUAGE PATHOLOGY OR RHEUMATOLOGY OR PEDIATRICS OR OTORHINOLARYNGOLOGY OR DENTISTRY ORAL SURGERY MEDICINE OR TRANSPLANTATION OR ALLERGY OR EMERGENCY MEDICINE OR INTEGRATIVE COMPLEMENTARY MEDICINE OR GERONTOLOGY OR ANDROLOGY) AND DOCUMENT TYPES: (ARTICLE OR PROCEEDINGS PAPER OR REVIEW) Timespan: All years. Indexes: SCI-EXPANDED, SSCI, A&HCI, CPCI-S, CPCI-SSH, BKCI-S, BKCI-SSH, ESCI, CCR-EXPANDED, IC. |
In summary, there has been a significant and exponentially increase in yearly publications involving AI, machine learning, and deep learning over 30 years. Radiology is the leading speciality in terms of volume of yearly publications and overall citations, followed by psychiatry and neurology. Extensive collaboration between radiology, psychiatry, neurology, and computer science/engineering was noted. These results provide an objective measure of relative involvement in AI research for multiple medical specialties.
Ethics approval: Not required
Conflicts of interest: None declared
Funding: Not required.
Provenance: Internally reviewed.
Corresponding Author
Professor G Dwivedi PhD MD MRCP FRACP FASE FASC, Wesfarmers Chair in Cardiology, address as above. Email: girish.dwivedi@perkins.uwa.edu.au
APPENDIX
Appendix: Publication results for all assessed specialties. The percentage of total publications exceeds 100% as each paper could involve more than one specialty.
Specialty | Total Count | Percent of total publications |
---|---|---|
Radiology | 783 | 0.33 |
Psychiatry | 406 | 0.17 |
Neurology | 287 | 0.12 |
Paediatrics | 239 | 0.1 |
Cardiology | 166 | 0.07 |
Oncology | 162 | 0.07 |
Ophthalmology | 155 | 0.07 |
Genetics | 149 | 0.06 |
General Surgery | 146 | 0.06 |
Radiation Oncology | 133 | 0.06 |
Public Health | 117 | 0.05 |
Neurosurgery | 116 | 0.05 |
General Medicine | 89 | 0.04 |
Urology | 80 | 0.03 |
Nuclear Medicine | 79 | 0.03 |
Gastroenterology | 68 | 0.03 |
Intensive Care | 66 | 0.03 |
Immunology | 63 | 0.03 |
Anaesthetics | 59 | 0.02 |
Otolaryngology | 51 | 0.02 |
Pulmonology | 47 | 0.02 |
Orthopaedic Surgery | 46 | 0.02 |
Obstetrics and Gynaecology | 45 | 0.02 |
Rehabilitation Medicine | 45 | 0.02 |
Endocrinology | 36 | 0.02 |
Dermatology | 35 | 0.01 |
Emergency Medicine | 35 | 0.01 |
Haematology | 33 | 0.01 |
Infectious Disease | 30 | 0.01 |
Cardiothoracic Surgery | 29 | 0.01 |
Rheumatology | 26 | 0.01 |
Addiction Medicine | 25 | 0.01 |
Geriatrics | 25 | 0.01 |
Nephrology | 25 | 0.01 |
General Practice | 23 | 0.01 |
Trauma | 17 | 0.01 |
Paediatric Surgery | 14 | 0.01 |
Sleep Medicine | 12 | 0.01 |
Maxillofacial surgery | 10 | 0 |
Occupational Health | 9 | 0 |
Palliative Care | 6 | 0 |
Plastic Surgery | 5 | 0 |
Vascular Surgery | 3 | 0 |
Sports Medicine | 0 | 0 |