Introduction
The term artificial intelligence (AI) was first coined in 1956 and refers to the study and design of intelligent agents.1 Significant advancements have been made in AI over the last decade, and it now has the potential to impact almost all aspects of human life, especially in medicine. A recent editorial suggested that AI in healthcare is ‘within touching distance’,2 and it has become possible to imagine sophisticated AI algorithms providing real-time analysis and personalised recommendations at point of care.2 Despite this, few AI technologies are in current clinical use, and the translation of technical successes into clinical practice will be a significant challenge.2 Much hyperbole surrounds AI technologies, but high-quality research is emerging and the potential for impact cannot be ignored.2
Early decision support systems were often built using manually programmed rules that encoded expert knowledge.3,4 These rule-based systems achieved some initial success, but the knowledge was fragile and many aspects of knowledge can be difficult to represent in written rules. Modern approaches make use of machine learning (ML) in preference to defined rules. ML is a subfield of AI research that has been defined as “…a set of methods that automatically detect patterns in data, and then utilise the uncovered patterns to predict future data or enable decision making under uncertain conditions”.5 Many popular ML algorithms were invented decades ago but they have since become powerful and capable due to increases in computing power, storage, and in the size of datasets produced by digitisation of information.4 Deep learning (DL) is in turn a sub-category of ML that involves training neural networks. It has become popular in recent years, benefiting again from increases in computational power and the growing availability of large datasets. DL uses multiple layers of non-linear processing units to represent complex and abstract concepts in terms of a hierarchy of simpler concepts.3,4 Such representations are not designed by human experts, but are instead learned through “training” the machine. DL models can have many layers of processing, with billions of connections between units.3 DL is the technology behind many recent medical AI success stories. For example, DL algorithms have achieved human or superhuman level performance in multiple narrow domains including electrocardiogram arrhythmia detection, pneumonia detection on chest x-rays, detection of diabetic retinopathy, and classification of skin cancers.6-9 DL has also been used to reveal relationships that are difficult or impossible for a human observer, such as accurately predicting patient age, gender, smoking status, HbA1c, systolic blood pressure, and risk of major adverse cardiac event from only a retinal fundus image.10 Global publications on AI have increased exponentially in the last decade (Figure 1).
Australia’s share of world health research is increasing.11 A 2016 report used bibliometric analysis to estimate that Australia’s share of world health research in 2012 was 3.8% and had increased only modestly from 2.5% in 2002.11 However, the Australian Society for Medical Research (ASMR) has expressed concerns over a dramatic erosion of Australia’s health and medical research workforce.12 A large proportion of Australia’s publications (39.6% in 2012) are supported by funding from the National Health & Medical Research Council (NHMRC), but due to static government investment in the NHMRC and falling grant funding rates, 670 full-time equivalent research positions were lost between 2013 and 2015 inclusive.12 The impact of this reduction on Australian AI research is unknown. A 2017 report assessed AI maturity in seven countries and found that Australia was least likely to have plans to deploy AI and most likely to report absence of AI-related skills.13 In the “Australia 2030 plan” by Innovation and Science Australia the prioritisation of the development of advanced capability in AI and ML was recommended.14
We performed a bibliometric analysis in order to assess if the quantity and quality of scientific publications on medical AI in Australia are comparable with other publishing countries.
Methods
The SCOPUS database was accessed on 24 March 2018 and searched for all articles containing the words “artificial intelligence” OR “machine learning” OR “deep learning” in title, abstract, or keyword, and published in the subject area “Medicine” between 1 January 2008 and 31 December 2017 (see Box). The source was limited to journals and conference proceedings, and document type was limited to article or conference paper. Conference proceedings are an important method of publication in computer science. The location of authors for each country was obtained through identifying their affiliation, and records with no affiliation information were removed. Records with affiliations for more than one country were counted as a publication for each country. Results were reported as total publications per year and publications per country

The ten countries with the most publications were compared in more detail. Publication quality was assessed by the total number of citations recieved per year and average citation rate per paper. Citation rate was calculated by dividing the number of citations a paper received up to March 2018 by years since publication. To account for differences in country population and economic development, publication counts were also expressed per capita and by gross domestic product (GDP) were calculated. Population and GDP (US dollars) information were obtained from the World Bank Group.15 Data were not available for 2017. Bibliometric analysis was performed on Microsoft Excel.
Statistics p-values were calculated using the two-sided chi-squared test for proportions and two-tailed t-tests for means.
Results
Output of AI papers
The initial search returned 13,903 citations. Of these, 295 with no affiliation information were removed, leaving 13,608 records for analysis, of which 9896 were articles and 3712 were conference papers. The 10 countries with the highest number of publications accounted for 85.3% of all publications of AI in medicine over the ten-year period (Table 1). Authors from the United States consistently published the most papers each year and accounted for the largest proportion (33.4%) of total world publications on AI. China at second place accounted for 11.6%. From 2016 to 2017 there was a 50% increase in the number of worldwide publications. Especially notable were the increases in output from China (2.5-fold), India (1.8 -old), Australia and the United States (both 1.5-fold). The 470 medical AI papers by Australian authors accounted for 3.4% of global output.

Citation rates
Papers from the USA achieved the highest number of citations (67,494), followed by China (21,473). Australian papers received 6604 citations (Table 2). However, there was no significant difference in average citations per paper between any of the top publishing countries, including Australia (Table 3).

Publication normalised on population and GDP
From 2008 to 2016, Canada had the highest average number of publications (2.07) per 1,000,000 population, followed by Australia (1.86) and the United Kingdom (1.57) (Table 4). These differences were not statistically significant. India (0.03) and China (0.09) had the lowest number of publications per million population (Table 4). Australia had significantly more publications per million population per year than the United States, China, Germany, India, Italy, France and Spain. (Table 4).

Canada also had the highest number of publications per unit (US$10 billion) of GDP (0.68), followed by India (0.63) and the United Kingdom (0.58). The value for Australia was 0.46, not significantly different from the top three countries (Table 5). Publications per GDP were significantly lower than the Australian rate for the US, China, Germany, and Italy. Since 2008, publications per capita have increased for nine of the top-10 countries (except France – Table 4), and publications per GDP have also increased for nine of the top-10 countries (except China – Table 5).
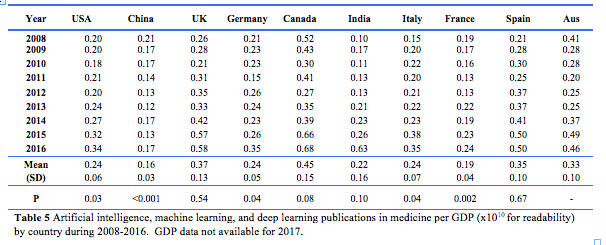
Discussion
World publications relating to AI in medicine have increased steadily over the 10 years to 2017, with a notable acceleration during the most recent quinquennium (Fig. 1). This is likely to correspond to a broader contemporaneous increase in AI capability in computing power, methodology (e.g. DL methods), size of medical data sets, and digitisation of medical records. Nine of the ten countries studied published more in 2017 than any other year since 2008.

The USA is the leader in terms of absolute publication volume and impact. This is to be expected given its population and level of development. In 2015, the US government invested approximately US$1.1 billion in AI related technology.16 However, China demonstrates the highest rate of recent growth. This is in line with their recent AI development plan which stated their aim to become the world leader in AI by 2030. China has published plans for a core AI industry on a scale exceeding the US (at US$150 billion).17 The plan has been supported by significant investments, including US$2.1 billion to build a technology park that is entirely dedicated to the development of AI technologies.18 In late 2017, Alibaba (a Chinese e-commerce company) announced investment of US$15 billion in AI research, and Baidu (Chines internet technology company) made a similar announcement in 2016.19 These projects complement corresponding investments by Google, Amazon and Apple in the US.19 With such significant government and private interest and investment in the development of AI technologies, it is likely that growth in AI publications in medicine will continue to increase, especially in China and the United States.
Australia has the lowest population (24 m) of the top 10 countries by a substantial margin, having half the population of the second smallest country (Spain, 46 m) in the list and less than 2% of the largest (China 1378 m). These facts help explain why output per year in Australia when adjusted by population is ranked 3rd of 10 behind Canada and the United Kingdom, but the difference amongst these countries was not statistically significant.
This review has a number of limitations. The 13,608 search results were not read in full and despite best efforts to ensure an accurate and thorough search, some irrelevant results may have been included. Only the SCOPUS database was searched due to the ability to extract citation information. While SCOPUS is a large database with extensive Medline coverage, some journals with relevant articles may not be indexed. Due to the large number of available machine learning techniques, some relevant results are likely to not have been returned under our the search strategy due to articles specified different keywords. It is likely that our search strategy underestimates the true published volume of global medical AI research. However, our aim was to provide a general high-level overview to compare research output between countries. Using a small number of very general terms allows capture of a large number of relevant articles and the assumption was made that trends observed in the large volume of records obtained are likely to be representative. In addition, the review also does not assess changes in popularity of machine learning techniques over time, and publications from 2008 are likely to be using different techniques than those from 2017. Population and GDP data were only available up to 2016, so we could not calculate normalised values for the final year 2017. We assess research output as a proxy to represent an aggregate of private and public interest, funding, prioritisation, and talent. Citations are a popular but imperfect proxy for research quality and impact, and publication rate per capita and GDP are imperfect measures of research priority and productivity.
Conclusion
There has been significant worldwide growth in publications on AI, ML, and DL in medicine over the last 10 years. This trend is likely to continue as AI technologies become increasingly capable and integrated into the practice of medicine, and AI research receives further prioritisation and investment.
Australian authors contributed 3.4% of the worldwide publications of AI research in medicine over the 10-year time-frame of this review. When publications are assessed by average citation rate or adjusted by country population, Australia’s contribution to AI research in medicine was comparable in quantity and quality to other top publishing countries. Significant and ongoing future investment is likely required for this to continue.
Provenance: Externally reviewed
Conflict of Interests: None declared
Funding: Not required
Ethics approval: Not required
Corresponding Author: Dr Jonathan E Stewart, Department of Emergency Medicine, Armadale Health Service, PO Box 460, Mount Nasura WA 6112, Australia. Email: Jonathan.Stewart@health.wa.gov.au